π² Comprehensive AI measurement systems
AI data and trends for business leaders | AI systems series
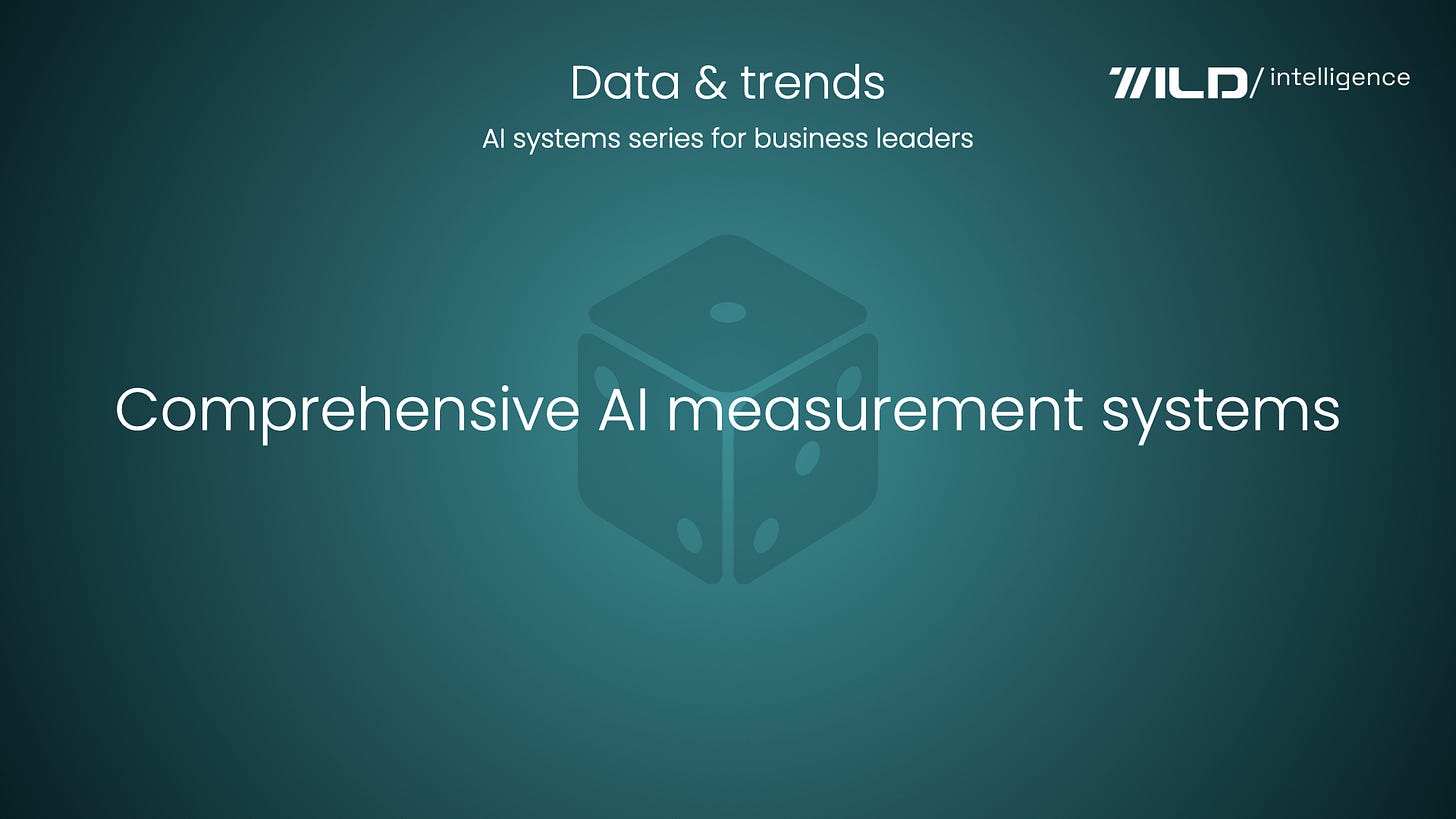
Hello,
Small reminder: this is the fourth post of a new series in the data and trends section.
The new series presents another angle, slightly different from the previous series that seeded the TOP framework1 and serves as the building block of our vision of AI safety implementation.
In this new series, we focus on more advanced topics in subsequent weeks, where we'll delve deeper into specific measurement methodologies and implementation strategies.
I believe this series will contribute significantly to the ongoing development of robust AI safety practices.βYael.
Previous posts from the series:
Comprehensive AI measurement systems
The increasing complexity and pervasiveness of AI systems necessitate the development of comprehensive measurement systems that provide real-time insights into performance, safety, and ethical considerations. These systems are crucial for ensuring accountability, transparency, and responsible AI deployment.
Building integrated measurement dashboards:
Integrated measurement dashboards provide a centralized view of key AI metrics, enabling stakeholders to monitor system performance and identify potential issues. These dashboards should:
Aggregate data: Collect data from various sources, including model performance metrics, safety indicators, and user feedback.
Visualize metrics: Present data in clear and intuitive visualizations, such as charts, graphs, and heatmaps.
Customize views: Allow users to customize dashboards to focus on relevant metrics and insights.
Real-time monitoring systems:
Real-time monitoring systems provide continuous feedback on AI system performance, enabling rapid detection and response to anomalies.
These systems should:
Track key metrics: Monitor critical metrics, such as latency, throughput, error rates, and safety indicators.
Implement alerting mechanisms: Trigger alerts when metrics exceed predefined thresholds.
Provide real-time analysis: Enable users to analyze data in real-time to identify root causes of issues.
Trend analysis and reporting:
Trend analysis and reporting provide insights into long-term patterns and changes in AI system performance. This involves:
Historical data analysis: Analyzing historical data to identify trends and patterns.
Generating reports: Creating reports that summarize key findings and recommendations.
Forecasting future trends: Using statistical methods to forecast future trends and potential risks.
Alert systems and thresholds:
Alert systems and thresholds are essential for detecting and responding to critical events on time. This involves:
Defining thresholds: Establishing thresholds for key metrics that indicate potential issues.
Implementing alerting mechanisms: Triggering alerts when thresholds are exceeded.
Prioritizing alerts: Prioritizing alerts based on severity and impact.
Future-proofing measurement systems:
Future-proofing measurement systems involves designing systems that adapt to evolving AI technologies and safety standards. This includes:
Modular design: Designing systems with modular components that can be easily updated or replaced.
Standardization: Adopting standardized metrics and protocols to ensure interoperability.
Scalability: Designing systems that can scale to accommodate increasing data volumes and complexity.
Real-world case studies:
Companies developing autonomous vehicles utilize comprehensive measurement systems to monitor sensor data, model performance, and safety indicators in real-time.
Financial institutions employ AI monitoring systems to detect and prevent fraud, ensuring the security and reliability of their AI-powered systems.
Healthcare providers use AI measurement dashboards to track patient outcomes and monitor the performance of AI-powered diagnostic tools.
By implementing comprehensive AI measurement systems, organizations can ensure the safe, reliable, and responsible deployment of AI technologies.
To address this, business leaders must consider:
How are you ensuring that your comprehensive AI measurement systems capture technical performance metrics and effectively translate those metrics into actionable insights for diverse stakeholders, including non-technical executives and regulatory bodies, to facilitate informed decision-making?
Given the dynamic nature of AI models and evolving safety standards, what strategies are you implementing to future-proof your measurement systems, ensuring they remain adaptable and relevant as AI technologies advance and regulatory landscapes shift?