📨 Weekly digest: 12 2025 | The subjective side of "better": How *do* we truly evaluate generative AI's real-world impact?
Moving past metrics: Finding meaning in AI's evolving capabilities | AI this week in the news; use cases; tools for the techies
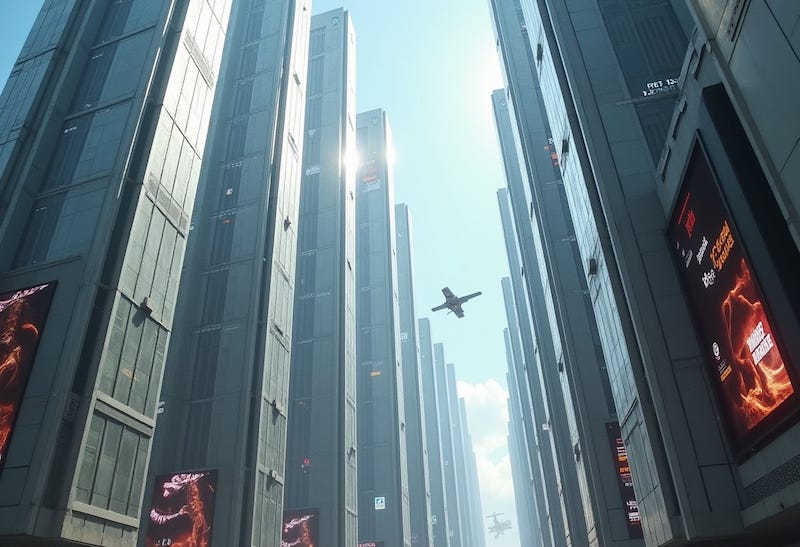
👋🏻 Hello legends, and welcome to the weekly digest, week 12 of 2025.
Navigating the whirlwind of generative AI advancements can feel like chasing a moving target.
Every week brings a new model, a fresh approach, and another tool to explore.
"Have you tried o1 Pro? Phi 4? Midjourney 6.1?" the questions echo, and you're left wondering: How do you truly measure progress?
Benchmarks offer a starting point, but their real-world relevance is often debated. A text file of logic puzzles might serve as a personal metric, but it still doesn't reveal the nuanced improvements in practical applications.
The most direct approach is to test these models within your own workflows.
Does this model genuinely perform better? Here, however, we encounter a fundamental challenge: the nature of "better" varies drastically depending on the task.
Some tasks exist within a spectrum of subjective quality. Consider creative endeavors like generating images with Midjourney.
Versions 3, 4, 5, and 6.1 of the same prompt yield visually distinct results, each with its own appeal. "Better" becomes a matter of personal preference.
This is where generative AI thrives, offering a playground for creative exploration.
Then, there are tasks where errors are easily identifiable and correctable. Asking ChatGPT for a draft email or cooking suggestions might produce inaccuracies, but these are readily spotted and fixed.
This explains generative AI's early and strong product-market fit in software development and marketing. Mistakes in code or marketing copy are often discernible, and the absence of a single "right" answer allows for iterative refinement.
We’ve always likened the previous wave of machine learning to "infinite interns." You can delegate numerous tasks, knowing some results will require revision, but the overall efficiency gain is substantial. This remains true for generative AI, particularly in automating tedious, time-consuming tasks that traditional software struggles with.
However, a critical limitation emerges when dealing with tasks requiring absolute accuracy. These are the "right or wrong" scenarios, where a mistake carries significant consequences.
Consider tasks like legal document analysis, medical diagnosis, or financial data analysis. In these domains, a single error can have severe repercussions. If I'm not an expert and lack the underlying data, verifying the LLM's output becomes as time-consuming as doing the task myself.
To bridge this gap, emerging strategies like retrieval-augmented generation (RAG) and fine-tuning on domain-specific data are crucial. RAG enhances LLMs' ability to access and utilize external knowledge, improving accuracy. Fine-tuning tailors models to specific domains, minimizing errors in specialized tasks.
Furthermore, user feedback is an important part of the improvement of LLMs. User feedback helps developers understand which areas of the model need improvement.
Looking ahead, the evaluation of generative AI must evolve beyond simple benchmarks.
We need metrics that assess nuanced accuracy, reliability, and practical utility improvements. Only then can we fully harness the potential of these powerful tools while mitigating the risks associated with their limitations.
What do you think?
I am looking forward to reading your thoughts in a comment.
Yael.
This week’s Wild Pod episode
Wildfires with Google, gaming with Roblox, hardware with Nvidia, Adobe revealed AI-driven enterprise, and Palantir
Stay ahead of AI innovation: your weekly Wild Intelligence brief
Yael on AI:
Sharing personal views and opinions on global advancements in AI from a decision leader perspective.
The open source imperative: winning the AI race through collaboration, not control
🦾 AI elsewhere on the interweb
“we trained a new model that is good at creative writing (not sure yet how/when it will get released). this is the first time i have been really struck by something written by AI; it got the vibe of metafiction so right.”—Sam Altman says OpenAI has trained a model that can write better fiction, as part of the general push towards ‘creativity’. And yet, his example seems so predictable. [LINK]
CoreWeave inks $11.9 billion contract with OpenAI ahead of IPO—. Is this a tech company or an SPV? [LINK]
Meta’s fact-checking replacement launches [LINK]
Fast access to our weekly posts
📌 AI case study: Apple's AI lag: A race against time in the era of intelligent technology?
🎲 Product change impact analysis
🎯 How to build with AI agents | Staying ahead of the curve: Monitoring emerging trends in AI
📮 Maildrop 18.03.25: Domain specialization in LLMs, part 3/3
🚀 Explainable AI (XAI): Illuminating the decision-making processes of AI [Week 6]
🚨❓Poll: In the context of open governance, how can we ensure AI-driven decision-making processes are both transparent and auditable to the public?
Previous digest
📨 Weekly digest: 11 2025 | AI-generated content explosion poses us significant challenges
👋🏻 Hello legends, and welcome to the weekly digest, week 11 of 2025.
📨 Weekly digest
Thank you for being a subscriber and for your ongoing support.
If you haven’t already, consider becoming a paying subscriber and joining our growing community.
To support this work for free, consider “liking” this post by tapping the heart icon, sharing it on social media, and/or forwarding it to a friend.
Every little bit helps!